AI In Cybersecurity: Defending Against Advanced Threats
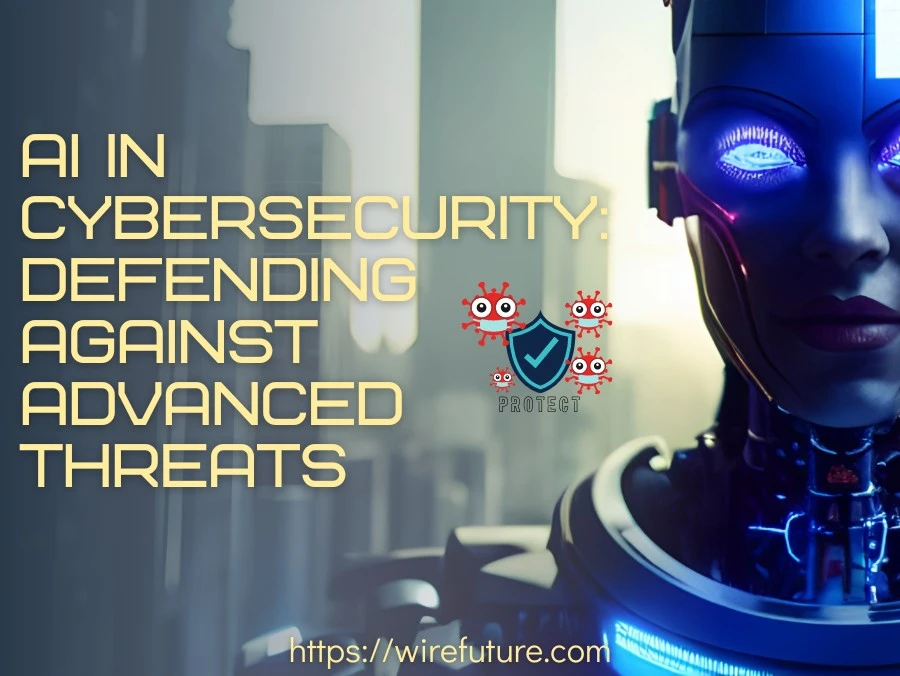
Complex and sophisticated cyber threats are emerging across the digital landscape. Hackers and crooks are continuously improving their methods, utilizing zero day exploits, ransomware and spear phishing to breach security systems. Evolution from simple viruses to multi-vector attacks illustrates the growing challenge for cybersecurity professionals. These advanced threats often require more sophisticated and proactive defense mechanisms, and traditional security measures often fail. To counter the proliferation of cyber threats, the use of AI in cybersecurity has been increasingly implemented in cybersecurity plans.
Artificial intelligence can easily analyze huge amounts of data at unprecedented speed and spot patterns and anomalies that human researchers couldn’t detect. AI-driven systems provide dynamic and adaptive threat detection and response giving organizations a strong tool to improve their cybersecurity posture. AI – enabled threat detection and incident response makes AI a key component of modern cybersecurity defenses.
Table of Contents
- The Role of AI in Cybersecurity
- Key Applications of AI in Cybersecurity
- Network Security
- Technologies Powering AI-Driven Cybersecurity
- Advantages of AI in Cybersecurity
- Challenges and Considerations
- Case Studies and Real World Implementations
- Best Practices on AI Integration in Cybersecurity
- Conclusion
The Role of AI in Cybersecurity
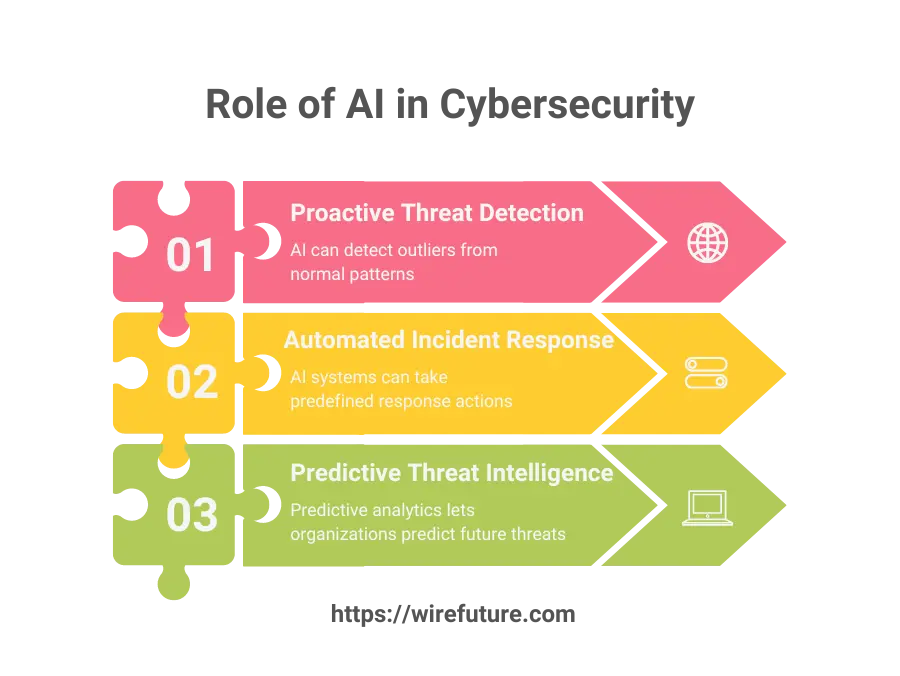
Proactive Threat Detection
AI in Cybersecurity specializes in identifying such threats before they occur. AI can detect outliers from normal patterns indicating a security breach by analyzing network traffic, user behavior and system logs in real time. This preventative approach enables organizations to respond to threats early and limit damage. Particularly, machine learning models can recognize novel attack vectors based on past incidents and corresponding new threat landscapes.
Automated Incident Response
One advantage of AI in cybersecurity is automation. When a threat is detected, AI systems can take predefined response actions like isolating affected systems, blocking malicious IP addresses or installing patches. This automated response capability shortens time to respond to threats and minimizes cyber incident impact. The repetitive and time-sensitive tasks AI can handle free human analysts to make more complex and strategic decisions that improve overall security operations.
Predictive Threat Intelligence
AI-driven predictive analytics lets organizations predict future threats based on historical data and trends. These insights help security teams prepare for attacks and strengthen defenses when needed. Predictive models may forecast different threat scenarios and help organizations allocate resources more effectively and develop proactive security measures. This forward-looking approach shifts cybersecurity from reactive to preventative discipline, decreasing the chance of successful attacks.
Key Applications of AI in Cybersecurity
Intrusion Detection Systems (IDS)
AI helps Intrusion Detection Systems detect malicious activities more accurately. Traditional IDS have high false positive rates that overwhelm security teams. AI-powered IDS distinguish legitimate from suspicious activities using advanced algorithms reducing false positives and increasing threat detection accuracy. These systems can learn from new data to respond to changing threats and issue more accurate security alerts by learning from new data.
Endpoint Protection
Endpoints such as PCs, smartphones and tablets are typical targets of cyberattacks. AI provides endpoint protection against malware and ransomware. Machine learning models examine file behaviors and patterns to discover malicious activities, even those not yet known to conventional antivirus software. AI-powered endpoint protection solutions offer real-time defenses against malware execution and propagation over networks.
Network Security
AI tracks and analyzes network traffic to discover unusual patterns which suggest a cyber threat. AI algorithms may spot anomalies like unusual data transfers, unauthorized access attempts or deviations from normal communication patterns. Analyzing such anomalies in real time may alert security teams of possible intrusions and prevent data breaches and unauthorized access.
User Behavior Analytics (UBA)
AI-driven user behavior Analytics (UBA) tracks and analyzes User activities to discover abnormal Behavior suggesting insider threats or compromised accounts. UBA systems create behavioral profiles of users based on typical activities and interactions. AI flags possible security incidents when deviations from these profiles are found. This approach is particularly effective for identifying threats beyond typical perimeter defenses such as insider threats or sophisticated social engineering attacks.
Technologies Powering AI-Driven Cybersecurity
Machine Learning (ML)
Many AI-driven cybersecurity applications rely on machine learning. It utilizes supervised and unsupervised learning methods to recognize and classify threats. Supervised learning uses labeled data to train models to recognize specific threats while unsupervised learning identifies patterns and anomalies in unlabeled data. Reinforcement learning can also be applied to form adaptive security measures that improve with time based on feedback from the environment.
Natural Language Processing (NLP)
Natural Language Processing (NLP) enhances cybersecurity by looking at text content to detect phishing attempts, social engineering attacks along with other text-based threats. NLP algorithms can decipher emails, messages and documents for suspicious content. This capability helps organizations identify suspicious emails and identify possible scams to increase security for text-based attacks.
Deep Learning
Deep learning employs neural networks to detect complex and subtle threats like zero-day exploits. These advanced models can analyze large datasets and thus detect sophisticated malware and intrusion attempts that traditional methods miss. The capability of deep learning to process enormous amounts of information and also learn from it enables it to identify brand new threats and also develop defences against emerging cyber threats.
Advantages of AI in Cybersecurity
Speed & Efficiency
AI enables faster detection and response to cyber incidents. Automated processes and real-time analysis shorten the time for identifying and mitigating threats thereby reducing potential damage and downtime. AI can process massive amounts of data quickly to provide timely and accurate security insights boosting cybersecurity efficiency.
Scalability
AI solutions are scalable and suitable for any organization size. They can handle huge data volumes and many endpoints with high performance. This scalability allows AI-driven security measures to grow alongside changing security requirements, protecting organizations as they grow.
Continuous Improvement
AI systems learn from new data and threats and enhance detection capabilities over time. This process of continuous learning allows AI to anticipate new threats and learn new attack methods. AI models can refine their algorithms and detect and react to threats by including feedback from real-world events.
Challenges and Considerations
Adversarial AI
Adversarial AI is where cybercriminals employ AI to create more sophisticated attacks. This includes creating malicious content that escapes detection or creating AI systems to automate cyberattacks. Defending against adversarial AI necessitates sophisticated countermeasures and adaptation to new attack strategies. Organizations must remain vigilant and invest in AI technologies that can combat adversarial techniques to maintain effective defenses.
Data Privacy
AI systems often need access to massive datasets containing sensitive information. Data access must be balanced with privacy concerns. Organizations must take adequate data protection and data privacy measures. This includes anonymizing data, access controls and regular audits to protect sensitive information while using AI for cybersecurity.
False Positives
AI prediction accuracy must be managed to reduce false alerts and burden on security teams. High false positive rates can cause alert fatigue and false threats to be masked. Organizations need to tune AI models so they are sensitive but precise enough to detect threats without overproducing false alarms. Regular model evaluation and validation is required for maintaining performance.
Case Studies and Real World Implementations
Phishing Detection
One large organization deployed an AI-based phishing detection system and was able to identify phishing emails better. It applied machine learning models to analyze email content and sender behavior, and the number of successful phishing attacks decreased by 85%. This case study shows how AI can improve email security and prevent social engineering attacks.
Threat Hunting
In the financial sector, a company used AI to discover advanced persistent threats (APTs). The AI system detected patterns associated with APT activities through network traffic and user behavior. Detecting these threats early averted a major data breach and bolstered its cybersecurity defenses. This real-world example demonstrates how AI can do proactive threat hunting and mitigation.
Best Practices on AI Integration in Cybersecurity
Seamless Integration
To leverage AI in cybersecurity effectively, organizations should combine AI solutions with existing cybersecurity measures. Seamless integration ensures AI systems complement and improve current defenses without interfering with operations. This entails linking AI capabilities with organization workflows, security policies and infrastructure to deliver a holistic cybersecurity approach.
Monitoring and Training Continually
Regularly updating AI models and monitoring their performances is essential for coping with new threats. Organizations should establish processes for regular model training and validation based on current threat intelligence and data. AI systems are monitored continuously to provide current protection against emerging cyber threats.
Collaborative Approach
Combining AI and human expertise is imperative for effective threat management. AI can handle routine and repetitive tasks while human analysts concentrate on strategic decision-making and complex threat analysis. Such collaborative approach leverages AI and human capabilities to improve overall cybersecurity effectiveness and resilience.
Summary of AI Role in Modern Cybersecurity
AI enables advanced threat detection, automated response and predictive intelligence in modern cybersecurity. Its ability to analyze huge datasets and recognize patterns makes cybersecurity an essential tool for defense against advanced threats.
Adaptation and Vigilance continue
The dynamic nature of cyber threats demands adaptation and vigilance. As attackers continue to refine their methods, organizations must adopt AI technologies and continuously improve their cybersecurity postures. AI as an enabler for cybersecurity is a crucial step towards preserving digital assets and information technology security.
Conclusion
AI enables advanced threat detection, automated response, and predictive intelligence in modern cybersecurity. Its capacity to analyze large datasets and detect complex patterns makes it indispensable in defending against advanced threats. The dynamic nature of cyber threats demands adaptation and vigilance. As attackers improve their methods, organizations need to leverage AI technologies and continuously improve their cybersecurity plans. AI in cybersecurity is an important step towards preserving digital assets and securing information systems.
As a custom software development company, we specialize in AI development and creating tailored solutions that enhance your cybersecurity capabilities. Our expertise ensures that your security systems are robust, adaptive, and ready to meet future challenges.
At WireFuture, we believe every idea has the potential to disrupt markets. Join us, and let's create software that speaks volumes, engages users, and drives growth.
No commitment required. Whether you’re a charity, business, start-up or you just have an idea – we’re happy to talk through your project.
Embrace a worry-free experience as we proactively update, secure, and optimize your software, enabling you to focus on what matters most – driving innovation and achieving your business goals.